Separate methods are required to analyze durations
mainly for three reasons: (i) durations generally follow a highly
skewed distribution – some subjects tend to have large and very large
duration whereas most will have relatively short; (ii) study of
duration requires follow-up and some subjects tend to be lost – they move
away, refuse to cooperate further, do not report for follow-up, etc; and (iii)
any follow-up is necessarily for a specified period and by the time you
terminate the study, some subjects may not have reached to the end-point
of interest – they are still alive, still feeding the child, still not
recovered, etc. In the last two cases, the duration is incomplete – you
only know that the duration is at least that much but do not know exactly
how much. For example, if you are observing infants for duration of breast
feeding and decide to follow-up 80 children for 6 months, it is possible
that 4 are lost midway to follow-up (drop outs) and another 12 still on
breast at the end of six-month period. Such values are called censored
values. Because of censoring, statistics such as mean can not be
calculated in a standard manner – neither the standard deviation. Thus a
separate method is required. A measure such as person-months is also not
applicable in this case; first, because of its inherent deficiency of
considering first month as important as say sixth month and second,
because censored values are treated same way as complete values.
Rather than the mean, the median survival duration is
generally used in survival analysis. The other parameters of interest
could be the entire ‘survival’ curve that is based on percentage of people
existing at various time points, survival rate at specific time such as
what percentage of children were on breastfeed at 4 months, and the
duration by which, say 90%, reach the end-point—such as out of 80
children, at what time only 8 are left on breast milk, or at what time
point, the survival is 10%. The methods of survival analysis adequately
provide for censored values that the usual methods fail to do. However
these methods depend on the type of censoring in the data as discussed in
a short while. Survival analysis is also used for estimating hazards as
explained later.
Besides modeling the survival pattern over a period of
time, the other objectives of survival analysis are (i) to
investigate factors that influence the duration of survival, (ii)
to compare two or more modalities for survival pattern, and (iii)
to estimate the future survival of individuals or groups with specified
features. Among many methods available for survival analysis, in this
communication we discuss only the more commonly used methods, namely, the
life-table, Kaplan-Meier, log-rank and Cox model. This might help those
who have duration data but are not comfortable in using survival analysis
methods due to intricacies. We are trying to present them in simple
language for our medical fraternity to increase awareness and to demystify
the methods. All the survival methods we discuss are nonparametric or
semiparametric and suit most types of duration. There might be isolated
examples where parametric models may be more accurate(7).
Survival pattern helps patients and physicians to
decide which treatment or health strategy to prefer and when. For example,
short term survival may be better (in terms of percentage) with one
regimen and long-term survival with another. For some conditions such as
peritonitis, deaths are rapid and the survival curve shows quick decline.
For others, such as kidney diseases, it may remain steady. The survival
patterns are lessons to health care providers and seekers about what to
expect in specific group of cases. The actual experience in individuals
can vary but not too much if the survival curves are valid and reliable.
Validity depends on correct recording of duration based on sharply defined
starting and end-points on representative sample of subjects, and
reliability depends on reasonable sample size.
Types of Censoring
Breastfeeding practically for all children starts from
the day of birth but consider duration of childhood disease that can start
at any age. When you start the study, if the child was already suffering,
the exact starting time of the disease may not be known. This is called
left censoring. Right censoring is the one discussed earlier where the
ending time is not known. This is very common. Both these censoring are
illustrated in Fig 1, including the completed observations
and incomplete values. Hollow squares show right censoring.
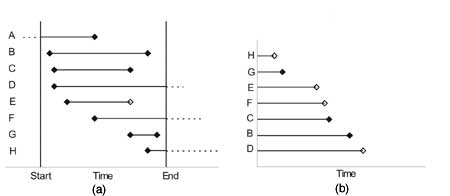 |
Fig. 1 (a)
Left and right censoring, (b) subjects ordered by available
durations excluding person A with left censoring. |
Third type of censoring occurs when you are asking
patients to report at monthly intervals or conducting home visits, say, at
every 3 months, to assess the status. In this case, only the interval will
be known but not the exact duration. This is called grouped-interval
censored data. In this setting also, right or left censoring can occur.
Left censoring is rare in duration studies and
inadvisable as it complicates the analysis. We exclude this from our
consideration in this communication. In addition, the general methods of
survival analysis require the following:
1. Survival pattern of those recruited early is the
same as those recruited late. Also, if the subjects are drawn from mixed
populations, all subgroups should have similar survival pattern. For
breastfeeding example, this means that breastfeeding duration should
generally follow the similar pattern in lower socio-economic segment as
in upper segment if the children in the study subjects are drawn from
both the segments. If pattern is not similar, the two segments should be
studied separately as two distinct groups.
2. Subjects with censored values should be random so
that they have same survival prospects as those with fully available
duration. For example, this means that those who are suffering seriously
should have the same dropout rate at any time point as those suffering
mildly. This is not easy to check since full data on censored values is
not available. External evidence, which may include clinical history and
biochemical parameters, may have to be gathered to validate this
requirement in case of doubt.
3. Quite often survival analysis is used to compare
two or more groups such as survival of patients in hospital care and
domiciliary care, or those on drug-1 versus those on drug-2. In our
breast-feeding example, the interest could be in comparing rural women
with urban women, or more educated versus less educated. Whenever two or
more groups are involved, the survival analysis methods require that
dropouts should be independent. Survival patterns of course can differ.
An editorial(8) has discussed how biased results may
have been obtained in survival analysis that does not check these
requirements. Precious few clinicians go into that many details.
Life-Table Method
Life table method of survival analysis is generally
used for grouped-interval censored data where the exact duration is
not known but only the interval is known. This method of data collection
is generally adopted when the number of subjects is really large and
periodic visits to the system are more cost-effective than continuous
observations. Usual life-table method assumes that the events occur
uniformly over the interval for subjects dropping out in that interval.
Probability of survival for each interval is obtained conditioned on
surviving the preceding interval. Survival function is obtained by
multiplication of the successive conditional probabilities.
Plot of survival function against the end-point of the
time interval, when joined by lines, is the survival curve. Although
software will give you the estimated median survival time, you can also
obtain approximate value of median by drawing horizontal line at 50%
survival rate and reading the corresponding survival time on the x-axis.
The survival curve can also be used to estimate other parameters of
interest such as 5-year survival rate, or the duration when 80% of the
subjects survive. All these are illustrated in Fig 2.
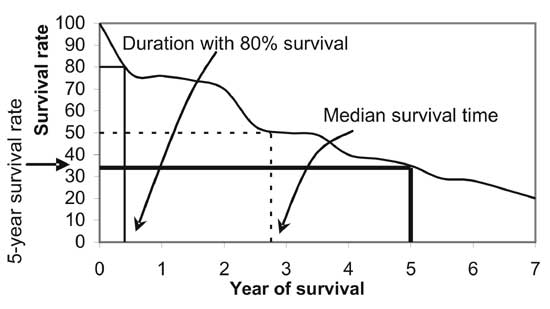 |
Fig. 2 Using survival curve for
obtaining various estimates. |
Statistical software will require that the censored
values are identified by a particular (software dependent) value, for
instance 0 for censored cases and 1 for fully known durations. The
software will estimate the (cumulative) survival probability at every time
point based on censored and complete values, and will plot the survival
curve of the type shown in Fig 3. Survival curve for samples
is a piecewise function where straight lines with constant slope are
connected at the ends of intervals.
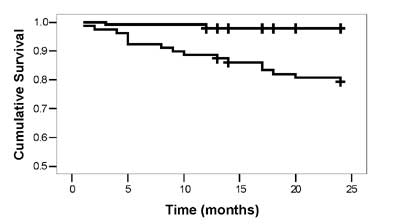 |
Fig. 3 Survival curves in two
groups under comparison (censored values are shown by + sign). |
Kaplan-Meier Method
Kaplan-Meier (K-M) method of survival analysis is used
when the subjects are continuously observed and exact duration of reaching
to the end-point or at the time of dropout is known. Dropouts are
considered in the analysis till the time they dropped out and after that
they are ignored since they are no longer considered at risk for the
end-point. Intuitively this looks most sensible thing to do for censored
values to avoid bias. Censored subjects should follow the same survival
pattern as those available.
Proportions survived of those at risk are obtained for
each unique time point at which an event occurred and the estimated
survival function (proportion surviving since the beginning) is obtained
again by sequential multiplication as for life-table method.
Kaplan-Meier method gives more accurate results than
life-table method. Interval censoring amounts to loss of some information
that affects the efficiency of the estimates. Thus, prefer continuous
observation of survival process so that exact time of survival or of
dropout is known for each subject unless n is really large and
periodic observations are cost-effective.
Plot of survival function against time is the survival
curve and can be used to estimate median survival time, quantiles and
other measures of the survival distribution. In this case, the survival
curve is a step function which moves down whenever deaths occur.
Log-Rank Test
The two methods discussed so far are for obtaining the
survival function that estimates survival probability for different time
points. Now consider the problem of comparing survival pattern in one
group with another such as in males and females, or in treatment and
control groups. If each time point has the same importance as another,
log-rank test is often used to compare the survival curves. In rare cases,
some investigators may be interested in giving more weight to the time
point where more number of subjects is available. Then log-rank is not
appropriate. Other methods such as generalized Wilcoxon rank-sum test are
used in this case(9). This method is also known as Gehan and Breslow test.
Since weighted by the number of subjects at risk, this method gives higher
weight to initial time points.
Log-rank method works on the same principle as
Kaplan-Meier and thus requires that survival duration is exactly available
for both groups. Expected deaths at each time point in either group are
obtained by following a procedure similar to the one followed for the
contingency tables. Total expected deaths for group-I and group-II are
compared with the total observed deaths in group-I and group-II,
respectively, and a chi-square value with 1 degree of freedom is obtained.
This is used to reject or not reject the null hypothesis of equality of
survival curves. For details of calculations, see Indrayan(10). Log-rank
method does not work for interval-censored data. Kim, et al.(11)
has proposed a variation of log-rank that may be applicable to
interval-censored data.
The log-rank test gives valid and easily interpretable
results when one survival curve is consistently higher than the other (Fig
3). This figure is for the data on different antibody levels
(Group I – where peak antibody level is below 15% and Group II – where it
exceeds 15%) in patients of renal allograft. The dataset in MSExcel is
available at http://www.medicalbiostatistics.com for those who want to
try. If they cross so that survival in one group is better up to a
particular time point, say 3 years, and worse thereafter, the log-rank
method loose validity.
Cox Model
A step further in survival analysis is to be able to
delineate the role of factors called covariates that affect duration of
survival. This is commonly done by Cox model. The covariates can be
continuous or categorical.
A basic requirement of Cox model is proportional
hazard. Hazard considers failure instead of survival. In a severe
earthquake, the hazard of death per hour in affected area is extremely
high relative to hazard of death in motor vehicle accidents.
Hazard naturally changes when one or more risk factors
are present. Hazard of discontinuing breastfeed steeply increases with
every passing month in working women relative to women at home. Theory of
relativity works in full measure here and hazard ratio is studied instead
of hazard alone. Hazard in women at home can be considered baseline and
hazard ratio in working women may be 3.1 at 3 months and 4.5 at 6 months.
This ratio could be different at different time points. Cox model requires
that the hazard ratio must remain same over the entire duration under
consideration, i.e., if hazard increases by 10% every month, this
should be so for working women as well as for women at home in our
example. Mathematically this means that logarithm of hazards are parallel
(Fig 4). This condition is popularly called proportional
hazards. A figure of the type in Fig 4 helps in case of one
covariate but not for multiple covariates. But the figure explains the
underlying requirement very well. The figure does not assess the
statistical significance of deviation from parallelism. One method to
check this is to test for interaction of log-time with each covariate by a
test such as Wald’s test. Check if your software has this provision.
Graphical plot should be adequate in most cases.
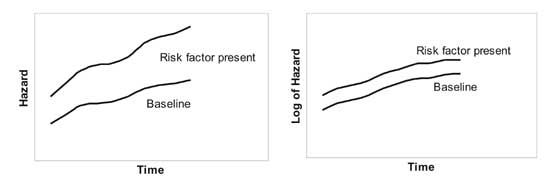 |
Fig. 4 Graphs showing meaning of
proportional hazards. |
In Cox model, the logarithm of hazard ratio is modeled
relative to a baseline as a linear function of covariates. The cumulative
survival function can be obtained from the cumulative hazard function
through a simple mathematical relation(12). The censored values are
adequately accounted for and contribute substantially to the model
building process.
Consider duration of survival of peritonitis cases
after surgery. This can be modeled to depend on the severity of disease at
the time of admission measured by APACHE score, age of the patient and sex
of the patient, beside other relevant factors. You can estimate the effect
of APACHE score on the duration of survival or test a hypothesis regarding
sex differentials in survival pattern. If the number of subjects in any
subgroup is extremely small or zero, you can get weird results such as
extremely large coefficients or large standard error. This can also happen
when covariates are strongly related, called multicolinearity. This
underscores the need to carefully scrutinize the data for suitability of
Cox model. The bottom line is the investigator himself who has to take all
the responsibility.
Risk factors in Cox model can be continuous such as
birth weight in breastfeeding example or categorical such as working
status of the woman. An important requirement of the usual Cox model is
that these risk factors should be time invariant. They should not change
during the period of the study. Whereas birth weight in this example
fulfills this criterion as it is a measurement before the onset of study
but working status may change during the six month period if that is the
period of the study. Age of the patient at onset of a disease is time
invariant but age during long follow-up is not. If the covariates are time
dependent, Cox model is still applicable but the method becomes
complex(13). Nonetheless, computer will go through its gyrations and come
up with an answer. This may leave an unenviable task for you to interpret
the coefficients since caution is required.
For further details of various survival methods and
related intricacies, see reference 9.
1. Hirve S, Ganatra B. A prospective cohort study on
the survival experience of under five children in rural western India.
Indian Pediatr 1997; 34: 995-1001.
2. Lee SJ, Ahn SJ, Lee JW, Kim SH, Kim TW. Survival
analysis of orthodontic mini-implants. Am J Orthod Dentofacial Orthop
2010; 137: 194-199.
3. Giashuddin MS, Kabir M. Breastfeeding duration in
Bangladesh and factors associated with it. Indian J Comm Med 2003; 28:
34-38.
4. Schaefer F, Marr J, Seidel C, Tilgen W, Scharer K.
Assessment of gonadal maturation by evaluation of spermaturia. Arch Dis
Child 1990; 65: 1205-1207.
5. Bajpai A, Kabra M, Gupta AK, Menon PSN. Growth
pattern and skeletal maturation following growth hormone therapy in growth
hormone deficiency: factors influencing outcome. Indian Pediatr 2006; 43:
593-599.
6. Sarin M, Dutta S, Narang A. Randomized controlled
trial of compact fluorescent lamp versus standard phototherapy for the
treatment of neonatal hyperbilirubinemia. Indian Pediatr 2006; 43:
583-590.
7. May M, Sterne J, Egger M. Parametric survival models
may be more accurate than Kaplan-Meier estimates. BMJ 2003; 326: 822.
8. Utley M, Gallivan S, Young A, Cox N, Davies P, Dixey
J, et al. Potential bias in Kaplan-Meier survival analysis applied
to rheumatology drug studies. Rheumatology (Oxford) 2000; 39: 1-2.
9. Hosmer DW, Lemeshow S. Applied Survival Analysis:
Regression Modeling of Time to Event Data. Wiley New York: Interscience;
1998.
10. Indrayan A. Survival Analysis. In: Medical
Biostatistics. IInd ed. New York: Chapman & Hall/CRC Press; 2008: pp.
620-622.
11. Kim J, Kang DR, Nam CM. Logrank-type tests for
comparing survival curves with interval-censored data. Comput Stat Data
Analys 2006; 50: 3165-3178.
12. Bewick V, Cheek L, Ball J. Statistics review 12:
Survival analysis. Crit Care 2004; 8: 389-394.
13. Fisher LD, Lin DY. Time dependent covariates in the
Cox proportional-hazards regression model. Annu Rev Public Health 1999;
20: 145-157.