There is lack of
information on the epidemiology of pediatric HIV in India with
lack of universal screening for HIV [1]. The HIV case load among
children remains unknown as the existing surveillance system in
India does not include children. The pediatric burden is
estimated/projected to be 6-7% of the adult HIV prevalence
[2,3]. Under-diagnosis of pediatric HIV was attributed to the
low sensitivity of screening tools at the community level with
suggested addition of parental factors to improve the positive
predictive value of the Integrated Management of Childhood
illnesses- HIV (IMCI-HIV) algorithm [4].
However, this algorithm is applicable to ages up to 5
years only. Children up to 18 years constitute 41% of the total
population [5]. Targeted screening of sick children seeking care
at healthcare facilities may be possible if the available
algorithm is modified for children of all ages. Identification
of HIV infection and linkage with anti-retroviral treatment
centers would improve child survival among HIV-infected
children, currently reported to be less than half of that in
adults [6]. This is an important step towards the highly
ambitious UN goal of ending AIDS as a public health threat by
2030 [7]. The present study attempted age-appropriate
modifications in the generic IMCI-HIV algorithm to create a
modified integrated algorithm, and used it to detect prevalence
of HIV in sick children 0 to 14 years old.
METHODS
This cross-sectional study was conducted in four ‘talukas’
(sub-district administrative regions) of Belgaum District,
Karnataka, India viz, Athani, Bailhongal, Gokak and
Belgaum, during 2013-2014. The study protocol was approved by
the ethics committee of St John’s Medical College and Hospital,
Bangalore in October, 2012. Informed consent was obtained from
caregivers of study participants.
Out of 628 available health care facilities, 113 where HIV
testing and care was available were listed by using the
following criteria: availability of a physician or a
pediatrician, and a case load of at least 30 patients in a
month. These 113 HCFs were further categorized into tertiary,
secondary and primary, and government and private facilities.
Ten facilities were selected by stratified randomization by a
person not involved in the study.
WHO generic IMCI screening algorithm [8] for HIV covered
children only up to 5 years of age. To extend the algorithm for
children up to >5-14 years of age in the study, an ICMR
constituted sub-committee suggested modifications in the WHO
generic IMCI- HIV algorithm. The sub-committee incorporated
features of Integrated Management of Adolescent and Adult
Illnesses [9], and a category of conditions common to children
of both age categories (0-5 and
>5-14) was named as ‘other clues for all children’ from
history suggestive of parental HIV infection. Few symptoms
applicable to adolescents were marked with an asterisk, denoting
using caution in application, due to the sensitive nature of the
questions.
The WHO generic algorithm was used
for ages up to 5 years. A child less than 5 years was referred
for HIV testing if two or more of the following signs were
present: pneumonia or severe pneumonia, persistent diarrhea or
severe persistent diarrhea, ear discharge (acute or chronic),
very low weight or severe malnutrition, oral thrush, parotid
enlargement, and generalized lymphadenopathy.
For 5-14 year ages the Modified
integrated algorithm was used.
A child 5-14 years old was referred for HIV testing, if
any one of the signs given in Box I, or any sign
from the ‘other clues’ given in Box II, was
present.
A child (aged up to <5 years)
satisfying a minimum of two criteria for their age category or
one criterion in ‘other clues’, and a child aged
5-14 years fulfilling a minimum of one criterion
in their age category or under ‘other clues’, was defined as
screen positive and
referred for age-appropriate HIV testing.
Forty eight physicians/pediatricians
posted at 10 healthcare facilities of the study area were
trained at the District training centre, to use the Modified
integrated algorithm. A post-training feedback evaluation and a
weekly visit by the research officer to provide technical and
supportive supervision and re-training was done. Tools such as
flow-charts, ready-reckoners and diagrams were provided and
displayed at each of these facilities.
Children who fulfilled the screening
criteria for HIV testing were referred for age-appropriate HIV
tests: Children <18 months by DNA PCR, Children >18 months
tested by ELISA. Trained counsellors provided pre-test
counseling to the parent/guardian. Test results were obtained
maintaining anonymity. HIV positive children were referred to
the appropriate healthcare facility for care, support and
treatment.
Data analyses:
Data were entered in Microsoft Office Access. Statistical
software SPSS IBM version 22.0 (Armonk, NY: IBM Corp.) was used
for univariate and multivariable logistic regression analysis
and statistical significance was tested using Wald statistics.
Overall fit of the model was tested using Hosmer-Lemeshow
Chi-square test with P-value >0.05 as the criteria for
good fit. HIV prevalence across categories was compared by
Chi-square test and mean values were compared by Student t
test. The following study variables were included for univariate
analysis: present status of pneumonia, persistent diarrhea in
the past 3 months, ear discharge, history of loss of weight,
repeated infection, painless swelling in the neck or armpit,
weight loss >10%, diarrhea more than one month, vaginal
candidiasis more than one month, presence of herpes simplex
ulceration more than one month, oral thrush (or) oral hairy
leukoplakia, esophageal thrush, multiple sexual partners, herpes
zoster presence, chronic skin or mouth condition, presence of
TB, WHO stage 4 condition for HIV children, developmental
regression, persistent seborrheic capitis, chronic lung disease,
unexplained death of parent, orphan child, blood transfusion
history, parent with HIV related illness, and parent with high
risk occupation. The variables that were found to be
statistically significant at P<0.05, were included for
multi variable logistic regression analysis.
A Classification and Regression Tree
(CART) [10,11] model using Salford Predictive Modeler (SPM)
software (evaluation version 8.0) was developed. CART is an
exploratory data analysis and builds a tree through recursive
partitioning in such a way that the data is successfully split
into increasingly homogenous subgroups. In the present analysis,
minimum number of observations for each terminal node was set as
³30.
Data collected from all 10 health facilities were included in
the model. Children with HIV serology negative were labelled as
HIV-negative and serology positive were labelled as
HIV-positive.
RESULTS
A total of 33342 children visited the 10 healthcare facilities
during the period 24 February, 2014 to 30 June, 2014. Among
them, 24342 were screened by trained field investigators and
confirmed by physician/pediatricians. Out of 527 sick children
screened positive by the modified IMCI-HIV algorithm, 509 (mean
age 6.8 y) consented and were tested with age-appropriate HIV
tests.
Overall HIV positivity in 0-14 years (n=509) was 19.1% (n=97)
(95% CI 15.7, 22.7); 5% in <5 years and 28% in
³5-14
years. Age and gender profile of the study population is given
in Table I. There was no significant difference in
the age distribution between the genders (P=0.3). The
mean age of boys was significantly higher than girls (P=0.005).
HIV prevalence in different taluks is shown in Table
II.
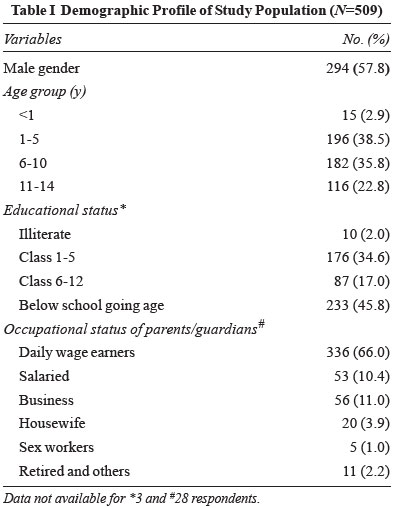 |
|
|
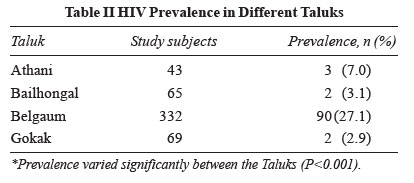 |
Only 14 out of 22 (64%) criteria in the modified IMCI–HIV
algorithm were found to be useful in identifying HIV infected
children. Univariate analysis identified eight variables
(persistent diarrhea, discharging ear, very low weight,
diarrhea, unexplained fever, orphan child, history of blood
transfusion and parents with HIV) as significantly associated
with HIV status. Final statistical model on multivariable
logistic regression yielded three significant variables
(unexplained fever, orphan child and parents with HIV); (Table
III). Using these three variables, correct classification of
negatives and positives was 90% and 95%, respectively.
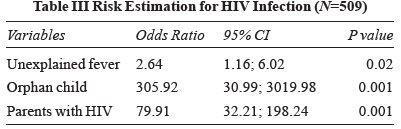 |
|
CART can statistically demonstrate which factors are
particularly important in a model or relationship in terms of
explanatory power and variance. Accordingly, these diagnostic
measures were compared between logistic and CART model (Web
Table I). It was observed that while identifying the
children of HIV parents and orphan, overall about 94% of HIV
children may be predicted correctly. The diagnostic measure was
compared between logistic and CART model which showed similar
sensitivity (94.8% and 94%), specificity (90% each) and
diagnostic accuracy (91.2% and 91%), respectively for
identifying the children with HIV parents and orphans. The area
under curve (AUC) is presented in Fig. 1. Apart
from HIV parents and orphan children, presence of unexplained
fever was captured as another significant variable in the
logistic regression analysis. However, diagnostic measures did
not vary significantly. Therefore, ‘parents with HIV’ and
‘orphan child’ are important with the order of priority scores
being 100% and 50%, respectively as emerged by CART analysis.
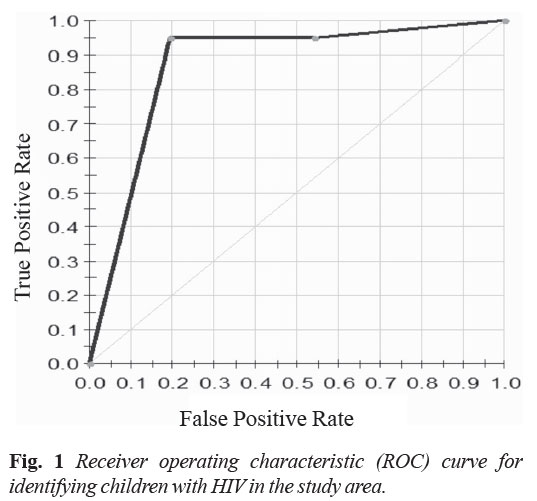 |
DISCUSSION
In this study, the WHO generic IMCI algorithm was modified by
addition of components from the Integrated Management of
Adolescence and Adult Illnesses and ‘other clues’ for suspecting
HIV infection in children
³5-14 years. A higher age-specific prevalence of HIV was
identified in
³5 -14 year
old children. The positivity rate among adults tested was
reported as 2.7 in Belgaum
in 2014. The prevalence in 0 - <5 year was similar as
estimated from adult HIV projections. Earlier studies [12,13]
have reported local adaptation of the WHO generic algorithm in
improving diagnosis of pediatric HIV; however, they were limited
to ages 0 - <5 years.
Most of the criteria in the Modified
integrated algorithm were useful in identifying HIV infected
children in this population. Unexplained fever more than one
month, being an orphan child and having parents with HIV were
the top three predictors of HIV test positivity, with high
percent correct classification of negatives and positives unlike
eight other common clinical features. Application of this
algorithm in similar settings else-where should take this into
consideration and make deletions in the algorithm based on
background prevalence.
The modified integrated algorithm can thus be further
modified and used to predict pediatric HIV infection in similar
settings.
In addition to a binary logistic
regression analysis, we performed a CART analysis [14], which is
mathe-matically identical to certain familiar regression
techniques, but presents the data in a way that is easily
interpreted by those not well versed in statistical analysis. In
a large public health project, CART is useful to present
preliminary data to clinicians or other project stakeholders who
can comment on the statistical results with practice knowledge
and intuition. This process yields a well informed and
statistically informative model than a singular clinical or
statistical approach. The results of CART model and logistics
regression model were in agreement and parents with HIV and
orphan child had high priority scores with high sensitivity and
specificity.
Limitation of the study was lack of
validation of the algorithm, which required blood sample
collection from children testing negative on the screening tool
(questionnaire) in healthcare facilities. Both practitioners and
patients resisted blood collection purely for research purposes.
A higher prevalence of HIV as reported in the study is expected
in the population of sick children. Considering the high
proportion (30-40%) of population belonging to 5-14 year age
group, policy makers should consider including HIV estimates in
routine surveillance until universal coverage becomes a reality.
We conclude that the modified
integrated algorithm developed by ICMR can be used as a
screening tool in the public and private health care settings to
detect pediatric HIV where universal screening for HIV is not
yet available/feasible. The important predictors of pediatric
HIV infection in settings with low prevalence and yet a high
burden, as in many LMICs are parent with HIV, being orphan and
unexplained fever
more than one month.
Contributor:
AS: coordinated the study, conceived the idea, wrote the
proposal, monitored study implementation, contributed to
statistical
analysis, provided critical inputs to the manuscript and
approved it; RW and RS were responsible for obtaining and field
level compliance for ethics, regulatory and financial approvals,
study implementation, data collection, and provided inputs for
revising the manuscript; PV: conducted advanced statistical
analyses, contributed to the manuscript, reviewed and revised
the manuscript; RSP: conducted data analyses, reviewed the draft
manuscript; and SI was responsible for regular guidance and
support, reviewed the manuscript.
Funding:
Indian Council of Medical Research.
Competing interests:
None stated.
What is already
known?
Addition of parental factors to the IMCI-HIV algorithm
improves the positive predictive value of the tool
applicable for children younger than 5 years in
low-resource settings.
What this study
adds?
A Modified Integrated Algorithm detected high prevalence
of pediatric HIV in sick children aged 0-14 years.
Unexplained fever for more than one month, a
HIV-positive parent and being orphan were predictors of
HIV infection among sick children in a
low-resource and high-burden setting.
|
REFERENCES
1. Sinha A, Nath A,
Sethumadhavan R, Isac S, Washington R. Research protocol for an
epidemiological study on estimating disease burden of pediatric
HIV in Belgaum district, India. BMC Public Health. 2016;16:446.
2. Department of Aids
control, Ministry of Health and Family Welfare of India,
Government of India, 2013: Annual report; 2012-13;P: 6.
3. National Institute of
Medical Statistics and National Aids Control Organization,
Government of India, 2012: Technical Report. India HIV
Estimates.2012; P: 39.
4. Chandra J, Sahi PK,
Gupta S, Gupta RA, Dutta R, Sherwal BL, et al. Addition
of questions on parental factors to the WHO (integrated)
management of childhood illnesses) IMCI-HIV algorithm improves
the utility of the algorithm for diagnosis of HIV infection in
children. J Trop Pediatr. 2019; 65:29-38.
5. Age structure and
marital status. Office of the Registrar General & Census
Commissioner, India,
Ministry of Home Affairs, Government of India. Available at:
http://censusindia.gov.in/Census_And_You/age_structure_
and_marital_status.aspx: Accessed on October 23, 2018.
6. UN Joint Programs on
HIV/AIDS (UNAIDS), Global Report: UNAIDS Report on the Global
AIDS Epidemic: 2010, December 2010, ISBN 978-92-9173-871-7.
Available at: http://www.refworld.org/docid/4cfca9c62. htm.
Accessed October 23, 2018.
7. Poku NK. HIV Prevention:
The key to ending AIDS by 2030. Open AIDS J. 2016;10:65-77.
8. World Health
Organization. (2000). Handbook: IMCI Integrated Management of
Childhood Illness. Geneva: World Health Organization. Available
at: http://www.who. int/iris/handle/10665/66533. Accessed
October 23, 2018.
9. Integrated Management of
Adolescent and Adult Illness (IMAI) modules. Treat 3 million by
2005. Available at:
http://www.who.int/3by5/publications/documents/imai/en.
Accessed October 23, 2018.
10. CART Classification and
Regression Trees. Salford systems. Available from:
http://www.salfordsystems.com/products/cart.
Accessed October 23, 2018
11. Breiman L, Friedman J,
Stone C. Classification and Regression Trees (Wadsworth
statistics/Probability). New York: Chapman and Hall/CRC; 1984.
12. lliff P, Ntozini R,
Nathoo K, Piwoz E, Moulton L; ZVITAMBO, Humphrey J. Making a
working clinical diagnosis of HIV infection in infants in
Zimbabwe. Trop Med Int Health. 2008;13:1459-69.
13. Bahwere P, Piwoz E,
Joshua MC, Sadler K, Grobler-Tanner CH, Guerrero S, et al.
Uptake of HIV testing and outcomes within a community-based
therapeutic care (CTC) programme to treat severe acute
malnutrition in Malawi: A descriptive study. BMC Infect Dis.
2008;8:106.
14. Cheng Z, Nakatsugawa M,
Hu C, Robertson SP, Hui X, Moore JA, et al. Evaluation of
classification and regression tree (CART) model in weight loss
prediction following head and neck cancer radiation therapy. Adv
Radiat Oncol. 2017;3:346-55.