|
Indian Pediatr 2019;56: 1017-1019 |
 |
Computer-aided Facial Analysis in Diagnosing
Dysmorphic Syndromes in Indian Children
|
Dhanya Lakshmi Narayanan 1,
Prajnya Ranganath1,
Shagun Aggarwal1,
Ashwin Dalal2,
Shubha R Phadke3
and Kaushik Mandal3
From Departments of Medical
Genetics, 1Nizam’s Institute of Medical Sciences, Hyderabad,
Andhra Pradesh; 2Diagnostics, Centre and DNA Fingerprinting
and Diagnosis, Hyderabad, Andhra Pradesh; and 3Sanjay Gandhi
Post Graduate Institute of Medical Sciences, Lucknow, Uttar Pradesh;
India.
Correspondence to: Dr Dhanya
Lakshmi Narayanan, Assistant Professor, Department of Medical Genetics,
Nizam’s Institute of Medical Sciences, Hyderabad , Andhra Pradesh,
India.
Email: [email protected]
Received: November 05, 2018;
Initial review: April 12, 2019;
Accepted: October 03, 2019.
|
Objective: To assess the utility
of computer-aided facial analysis in identifying dysmorphic syndromes in
Indian children. Methods: Fifty-one patients with a definite
molecular or cytogenetic diagnosis and recognizable facial dysmorphism
were enrolled in the study and their facial photographs were uploaded in
the Face2Gene software. The results provided by the software were
compared with the molecular diagnosis. Results: Of the 51
patients, the software predicted the correct diagnosis in 37 patients
(72.5%); predicted as the first in the top ten suggestions in 26
(70.2%). In 14 patients, the software did not suggest a correct
diagnosis. Conclusions: Computer-aided facial analysis is a
method that can aid in diagnosis of genetic syndromes in Indian
children. As more clinicians start to use this software, its accuracy is
expected to improve.
Keywords: Facies, Gestalt theory, Photographs.
|
M any of the genetic syndromes have distinct facial
features and recognizing a syndrome from facial gestalt is the first
step in diagnosis. Based on facial features, many a times, a geneticist
or a pediatrician is able to reach a possible diagnosis and order
appropriate tests for confirmation of the same. In many instances,
failure to identify a particular genetic syndrome results in a delay in
diagnosis and unwarranted investigations for the patients and families.
Memorizing facial gestalt of common syndromes is a cumbersome task and
is challenging for geneticists and pediatricians. With recent advances
in artificial intelligence, tools like Face2Gene (FDNA, Inc., Boston,
MA) have been developed, which aid clinicians recognize genetic
syndromes based on facial gestalt. Face2Gene utilizes deep convolutional
neural networks (DCNN) and compares a patient’s gestalt to its database
for syndrome suggestion [1]. Previous studies have shown superiority of
this computer-aided facial recognition in identifying genetic syndromes
in different populations groups [2-4]. Since the original software was
trained with photographs from Caucasian population and since the results
of the software can change based on ethnicity, it becomes important to
assess its utility in other populations. Our aim was to assess the use
of Face2Gene in accurately identifying proven genetic syndromes in
Indian children.
Methods
After obtaining informed consent from parents or
guardians, patients with recognizable facial dysmorphism and a proven
genetic diagnosis were included in this study from the records available
in the Genetics outpatient departments from two different centers in
India between January to June 2018. Institutional Ethics Committees
approved the study. Age, sex, anthropometry and other clinical details
were noted from the records. A minimum of two facial photographs (a
frontal and lateral, wherever available) were collected and uploaded to
the Face2Gene CLINIC app. No clinical details were added in the first
go. If the software provided the correct clinical diagnosis as one among
the first ten differential diagnoses, we considered it as a positive
result. If the correct diagnosis was not available in the first ten
diagnoses, then we added additional clinical information required and
modified the phenotype and assessed whether the software gave a correct
diagnosis. If the software was unable to provide the correct diagnosis
even after providing additional information, it was taken as a negative
result.
Results
Fifty-one patients (28 males, age 11 d-18 y) with a
facial phenotype and a proven genetic diagnosis were included in the
study. Fifteen (29.4%) children had chromosomal abnormalities or micro
deletion/ duplication syndromes, and rest had single gene disorders. A
positive result (correct diagnosis in the first ten differential
diagnoses) was obtained in 37 patients (72.5%); in 26 patients (26/37;
70.2%), the correct diagnosis was the first in the list. In 15 patients,
the correct diagnosis was not listed in the first ten diagnoses. Out of
these 15, for one patient when additional details were provided, the
software predicted the correct diagnosis as one among the predicted ten
diagnoses and hence that was considered as a positive result. Of the 14
patients for whom the software did not provide the correct diagnosis, no
suggestions were obtained for 8 patients. Web Table 1
summarizes the details of the patients and the prediction by the
software. We herein describe two representative cases to explain the use
of the software.
Patient 1: A 13-year-old female, who was the only
offspring of non-consanguineous parents, was brought for evaluation of
mental subnormality and primary amenorrhea. On evaluation, she had
facial dysmorphism with prominent metopic ridge, microcephaly and
borderline mental sub normality (Fig. 1a, 1b). MRI pelvis
showed hypoplastic uterus and ovaries. Her karyotype was 46,XX.
Chromosomal microarray was normal. Since she had facial dysmorphism, her
photograph was uploaded to Face2Gene, which predicted the diagnosis of
fetal valproate syndrome with high accuracy (Fig. 1c). On
revisit, details about maternal drug intake during pregnancy were
enquired to the grandparents who accompanied the patient. The mother was
on 1000 mg of sodium valproate during the entire course of pregnancy.
This suggests that the child most probably had fetal valproate syndrome,
though this could not be conclusively proven; hence, she was not
included in this cohort.
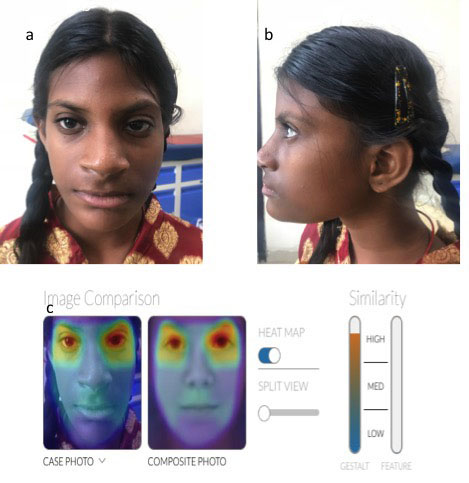 |
Fig. 1 (a) Frontal view of patient
with probable fetal alcohol syndrome showing high forehead,
prominent metopic suture, long thin upper lips, (b) Low set
ears; and (c) Heatmap showing high similarity of patient
photograph with that of Fetal Valproate syndrome.
|
Patient 2: A four-year-old female was evaluated
for developmental delay. She did not cry soon after birth. She had
facial dysmorphism and swelling in nape of neck at birth. She never had
any seizures. She had arched eyebrows, short neck, epicanthal folds and
bilateral iris coloboma (Fig. 2a, b, c). We
suspected chromosomal abnormality. But the software predicted it to be
Baraitser- Winter syndrome. Baraitser-Winter syndrome can be caused due
to heterozygous variations in ACTB or ACTG1 genes. Exome
sequencing in the proband identified a previously unreported
heterozygous variant in exon 4 of the ACTB gene (c.575T>G
p.Ile192Ser), located in a highly conserved nucleotide and amino acid
position, confirming the diagnosis of Baraitser-Winter Syndrome. The
same variant was absent in her parents indicating that the variant was
de novo.
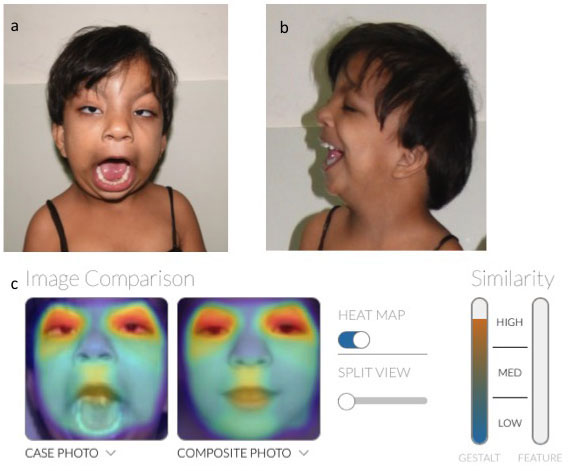 |
Fig. 2 (a,b): facial dysmorphism in a
child with Winter Baraitser syndrome; and (c) Heatmap showing
high similarity of patient photograph with that of fetal
valproate syndrome.
|
Discussion
In our study, the software was able to provide an
accurate clinical suggestion as the first option in 50% of patients. But
in some easily recognizable conditions like Turner syndrome, Waardenburg
syndrome and Wolfe Hirschhorn syndrome, the software was unable to
provide a diagnosis. Even though our study is descriptive and limited in
terms of the number of patients, results show that computer-aided facial
recognition for syndrome identification can be used for diagnosing
genetic syndromes.
Advances in computer vision and machine learning have
now aided in the development of softwares like Face2Gene, which enable
face recognition and thus syndrome diagnosis. Face2Gene is able to
compare 2D facial images to more than 300 syndromic phenotype models
[6]. Vanagaite, et al. [2] concluded that Face2Gene detection
rate was comparable to that of dysmorphologists in a study done on
facial recognition of Cornelia De Lange phenotype. A study on computer-
aided facial recognition of fetal alcohol spectrum disorders concluded
that software enabled better detection of individuals with fetal alcohol
spectrum disorders [3]. Though geometric morphometrics was used to
characterize Rubinstein Taybi syndrome [7], studies using computer-aided
facial recognition for syndrome diagnosis were lacking in India. To the
best of our knowledge our study is the first such attempt from India.
Deep phenotyping is also important in the next generation sequencing era
because it helps in classification of variants and ascertains their
clinical significance.
As more and more clinicians use the software, the
system can be trained to make more accurate diagnosis. Computer-aided
facial recognition has an important role in diagnosing genetic syndromes
and can be used as a tool by both pediatricians and geneticists.
Funding: None; Competing interests: None
stated.
Consent for publication of photographs has been
obtained from parents of included children.
What This Study Adds?
•
This study demonstrates the use
of computer-aided facial analysis in diagnosing syndromes in
Indian patients.
|
References
1. DeepGestalt – identifying rare genetic syndromes
using deep learning. Available from https://arxiv.org/abs/1801.07637.
Accessed November 1, 2018.
2. Basel-Vanagaite L, Wolf L, Orin M , Larizza L,
Gervasini C, Krantz ID, et al. Recognition of the Cornelia de
Lange syndrome phenotype with facial dysmorphology novel analysis. Clin
Genet. 2016; 89: 557-63.
3. Valentine M, Bihm DCJ, Wolf L, Hoyme HE, May PA,
Buckley D. Computer-aided recognition of facial attributes for fetal
alcohol spectrum disorders. Pediatrics. 2017; 140:6.
4. Vorravanpreecha N, Lertboonnum T, Rodjanadit R,
Sriplienchan P, Rojnueangnit K. Studying Down syndrome recognition
probabilities in Thai children with de-identified computer-aided facial
analysis. Am J Med Genet A. 2018;176:1935-40.
5. Hart T, Hart P. Genetic studies of craniofacial
anomalies: Clinical implications and applications. Orthod Craniofac Res.
2009;12:212-20.
6. Pantel J, Zhao M, Mensah M, Hajjir M, Hsieh T,
Hanani Y, et al. Advances in computer-assisted syndrome
recognition by the example of inborn errors of metabolism. J Inherit
Metab Dis. 2018;41:533-9.
7. Dalal AB, Phadke SR. Morphometric analysis of face in
dysmorphology. Comput Methods Programs Biomed. 2007;85:165-72.
|
|
 |
|